Physiologic determinants of serum galectin-3 in a general healthy population
Introduction
According to the 2017 statistics of the American Heart Association (AHA), acute myocardial infarction (AMI) remains the leading cause of mortality in the US, accounting for approximately one of every seven deaths (1). Even more importantly, patients surviving the acute stage of a heart attack have a risk of further illnesses or death that is between 1.5–15 times higher than that of the general population, and this has been especially attributed to the substantial risk of developing recurrent AMI, sudden death, heart failure or stroke. Beside the huge direct costs of AMI, which have been estimated at around 200 billion U.S.$ each year, the burden on the society and on the healthcare system of patients recovering from myocardial ischemia is dramatic, with as many as 1.346 million hospital discharges/year (0.4% of the resident U.S. population), 8.953 million physician office visits/year (2.9% of the resident U.S. population) and additional 0.480 million emergency department visits/year (0.15% of the resident U.S. population) of patients with a diagnosis of coronary heart disease (1). These concerning figures actually mirror an epidemiological burden that is not really different in other parts of the world, especially in the so-called “developing” countries (2).
The risk assessment of patients who have experienced an AMI is essential in clinical practice (3), and it is now currently advocated by many national and international organizations worldwide. A joint document of the North American society for Cardiovascular Imaging and the European Society of Cardiac Radiology clearly highlights that the use of imaging techniques (namely echocardiography, perfusion positron emission tomography and cardiac magnetic resonance) should be routinely performed after diagnosing AMI, for establishing both the short- and long-term prognosis of patients with ischemic myocardial injury (4). The most recent guidelines of the European Society of Cardiology (ESC) also recognize that the measurement of some selected biomarkers may provide prognostic information that is more reliable than cardiac troponins (cTns), although evidence is still lacking that routine measurement of any of these biomarkers may actually impact patient management (5). Despite this statement, recent evidence suggests instead that the measurement of some biomarkers may be an appealing perspective for stratifying the short- and long-term risk of morbidity and mortality after AMI or in those with heart failure. Accordingly, the current panel of available tests include biomarkers of cardiomyocyte necrosis such as cardiac troponin I (cTnI) and T (cTnT), biomarkers of post-infarction fibrosis such as galectin-3, growth differentiation factor 15 (GDF-15) or soluble suppression of tumorigenicity 2 (ST-2), along with biomarkers of cardiac stress such as natriuretic peptides (i.e., atrial natriuretic peptide, B-type natriuretic peptide, C-type natriuretic peptide, D-type natriuretic peptide and adrenomedullin) (6-9), or non-specific prognostic measures such as the red blood cell distribution width (RDW) (10). Among the various biomarkers of fibrosis, galectin-3 is one of those receiving major focus. Galectin-3 (also known as carbohydrate-binding protein 35 or Mac-2 antigen) is a 250-aminoacid protein with a predicted molecular weight of 26.2 kDa (11). Growing evidence suggests that this protein may promote fibrosis and cardiac remodeling, thus being currently considered also an important cause of arrhythmias (12).
Before any putative biomarker can be introduced into clinical practice, many important preliminary aspects needs to be assessed, including the evaluation of analytical and clinical performance, validation of personalized cut-offs, as well as identification of leading determinants of plasma or serum concentration (13). In a previous study based on 33 consecutive elderly patients (mean age 66 years) admitted to the observation unit of an urban emergency department, in whom a diagnosis of AMI could be ruled out, we observed that the serum concentration of galectin-3 was independently associated with RDW, but not with age, sex, serum bilirubin, serum glucose and renal function (14). Moreover, lower levels of galectin-3 were also found to be associated with successful aging (15). Different results were published in ensuing articles and based on other populations, thus including healthy individuals (16,17) and outpatients with renal failure (18).
Therefore, this original study was planned to identify the main physiological determinants of serum galectin-3 in a reference population of middle-aged healthy subjects, thus providing useful information for definition of more accurate (i.e., personalized) diagnostic thresholds.
Methods
Study population
The study population consisted of 44 healthy volunteers [14 males and 30 females; mean age, 47±11years; body mass index (BMI), 23.6±3.6 ], recruited from the personnel of the local laboratory. All the volunteers underwent a regular medical examination as part of standard hospital check-up. None of the subjects reported current use of medications known to significantly impair the biochemical profile. Blood was collected by venipuncture between 8:00–9:30 AM, from subjects at fast, into evacuated blood collection tubes containing no additives (Terumo Europe N.V., Leuven, Belgium). The venous blood samples were then centrifuged at 1,500 ×g for 15 min at room temperature and serum was used for biochemical testing. All subjects provided a written consent for being enrolled in this study. The evaluation was performed in agreement with the Declaration of Helsinki, under the terms of relevant local legislation, and was approved by the local Ethical Committee (University Hospital of Verona, Verona, Italy—SOPAV2, protocol number 35747; 25 July 2016).
Laboratory measurements
The concentration of serum cTnT was measured using Roche Cobas 6000 (Roche Diagnostics GmbH, Mannheim, Germany). Specifically, cTnT was assayed with a high-sensitivity (HS) method displaying a limit of blank and a 99th percentile of the upper reference limit (URL) of 3.0 and 14.0 ng/L, respectively (19). The concentration of serum galectin-3 was quantified using Vidas (BioMérieux, Marcy l'Etoile, France). The functional measuring range of this method is comprised between 3.3–100 ng/mL and the between-run imprecision was reported to be comprised between 3.9–4.5% (20). The concentration of galectin-3 in healthy individuals was found to be comprised between 5.3–18.0 ng/mL, with an URL of 16.1 ng/mL (20). Serum creatinine was assayed using the conventional alkaline picrate-based method, whereas albumin was measured with the bromocresol green assay. Both analytes were quantified on Roche Cobas 6000 (Roche Diagnostics GmbH, Mannheim, Germany). The renal function was finally reported as “estimated glomerular filtration rate” (eGRF), calculated according to the CKD-EPI (Chronic Kidney Disease Epidemiology Collaboration) equation (21). Finally, the complete blood count was performed on EDTA-anticoagulated whole blood, using Advia 2120 (Siemens Advia 2120, Diagnostic Solutions, Milan, Italy).
Statistical analysis
All results of laboratory measurements were finally reported as median value and interquartile range (IQR). The comparison among groups was performed using Mann-Whitney U test and ANOVA (one-way analysis of variance), whereas the potential associations among variables were analyzed with univariate analysis (Spearman’s correlation) and multiple linear regression analysis. To identify independent determinants of galectin-3, the variables found to be significantly associated with serum galectin-3 in univariate analysis (i.e., P<0.05) were then entered in multivariate regression after logarithmic transformation of values. The statistical significance was set at P<0.05. The statistical analysis was carried out with Analyse-it (Analyse-it Software Ltd, Leeds, UK) and MedCalc version 12.3.0 (MedCalc Software, Mariakerke, Belgium).
Results
The main physiological and biochemical characteristics of the study population are shown in Table 1. The serum concentration of galectin-3 ranged between 4.6 and 14.9 ng/mL (median value, 10.3 ng/mL; IQR, 9.0–11.4 ng/mL) in our reference healthy population, thus comprised within the 16.1 ng/mL URL formerly identified by Gruson et al. (20). The serum concentration of cTnT in all subjects ranged between 3.00-9.32 (median value, 3.02; IQR, 3.00–3.94), thus also comprised with the 14 ng/L URL (19). In univariate analysis, the concentration of serum galectin-3 was found to be significantly associated with BMI and eGFR, but not with age, sex, serum creatinine, serum albumin, serum cTnT and hemoglobin (Table 2). In multivariate analysis, the concentration of serum galectin-3 remained inversely associated with eGFR (Table 2, Figure 1), but not with BMI. The distribution of serum galectin-3 values according to quartiles of eGFR is shown in Figure 2. The median value of galectin-3 was found to be significantly different across quartiles of eGFR (ANOVA, P=0.046), and thus inversely associated with renal function. The 75th percentile of the URL increased from 11.2 ng/mL in the first quartile of eGFR, up to 13.3 ng/mL in the fourth quartile of eGFR. When a 12.0 ng/mL value of galectin-3 was used as a cut-off in our healthy population, subjects in the lowest eGFR quartile had a nearly 60% higher likelihood of displaying galectin-3 values above this arbitrary threshold (relative risk, 1.57; 95% CI, 1.01–2.46).
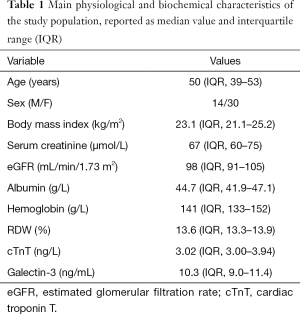
Full table
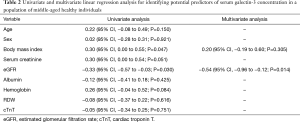
Full table
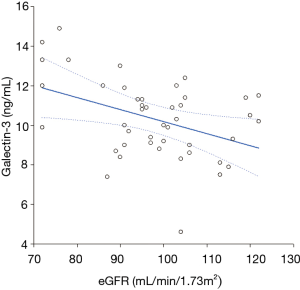
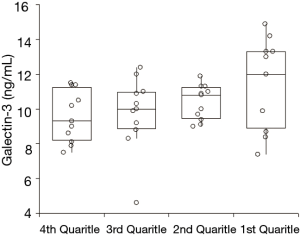
Discussion
The accurate risk stratification of patients with AMI is currently considered a clinical mainstay for reducing the short- and long-term risk of morbidity and mortality. According to this unavoidable aspect of managed care, all patients with AMI should undergo risk assessment with currently validated tools. The immediate risk stratification is conventionally performed using two of the most validated score systems, i.e., the GRACE (Global Registry of Acute Coronary Events) or TIMI (Thrombolysis in Myocardial Infarction) scores (22). The latter risk stratification tool, which is typically performed before or early after discharge, is most widely used to define the long-term prognosis after AMI. Among the other available tools, the HEART (history, electrocardiogram, age, risk factors and troponin) score is apparently one of the most accurate models for predicting MACE (major adverse cardiac event) and mortality in chest pain patients admitted to the emergency department (23). Despite a good predictive performance was proven for this model, its diagnostic accuracy is still suboptimal and can be significantly improved by incorporating some laboratory measures such as RDW (24). Notably, the recent study CLARITY-TIMI 28 (Clopidogrel as Adjunctive Reperfusion Therapy-Thrombolysis in Myocardial Infarction 28), which included 1258 patients (25), also showed that the incidence of cardiovascular death or heart failure at 30 days after an AMI could be significantly predicted by measuring cTnT [odds ratio (OR), 2.15; 95% CI, 1.75–2.63], ST-2 (OR, 1.76; 95% CI, 1.40–2.21), galectin-3 (OR, 1.50; 95% CI, 1.24–1.82) or GDF-15 (OR, 1.26; 95% CI, 1.02–1.55). After multivariable adjustment, the risk of heart failure was still predicted by ST-2 (OR, 2.68; 95% CI, 1.35–5.36) and galectin-3 (OR, 2.22; 95% CI, 1.04–4.71), emphasizing the crucial role of these biomarkers in management of these patients.
As for current evidence, the clinical significance of measuring galectin-3 in the risk stratification of AMI patients is supposed to considerably increase in the very next future (26), along with its predictable usefulness for predicting adverse outcomes in patients with heart failure (27). Before doing so, however, the main analytical and clinical characteristics of this biomarkers need to be deeply investigated and validated.
Taking together, the results of our investigation provide important clinical reflections. First, the lack of correlation that we observed between the serum values of galectin-3 and those of cTnT confirms previous published evidence (18), attesting that the two biomarkers actually target different metabolic pathways (i.e., cardiac remodeling and cardiomyocyte injury) and do not overlap. It is hence conceivable that whatever biomarkers-based algorithm for predicting AMI outcome should incorporate the measurement of both tests. A similar consideration can be done for RDW, wherein its lack of association with galectin-3 (Table 2) may allow their combination for predicting the risk of post-AMI complications in patients with or without heart failure.
A second important aspect that we have observed in our study is that serum galectin-3 values are virtually independent of age, sex, BMI, liver function and hemoglobin concentration, so the diagnostic cut-off in the general healthy population does not need to be partitioned for these physiological and biochemical determinants. Unlike these parameters, a significant, independent and inverse association was observed between serum galectin-3 values and renal function (Figure 1). Additionally, a gradual decrease of the eGFR was independently associated with gradually increased galectin-3 serum concentration (Figure 1).
Our findings on the relationship between renal function and serum galectin-3 confirm previous evidence. Gruson et al also found a strong, independent and inverse association between serum galectin-3 and eGFR in patients with heart failure (ρ=−0.41; P<0.001). A similar association was observed by Stoltze Gaborit et al. in elderly outpatients with heart failure (P<0.001) (18) and by Krintus et al. in a presumably healthy population (r=−0.16; P<0.001), although the correlation was no longer statistically significant after adjustment for age, sex, BMI, family history of premature coronary artery disease and smoking status (16).
With regards to age and sex, Stoltze Gaborit et al. observed that heart failure outpatients with galectin-3 values over the median serum concentration tended to be older than those with galectin-3 values below this threshold (i.e., 73 versus 68 years; P=0.010), whereas no difference was observed between genders (18). A similar finding was reported by Krintus et al., who found a significant correlation between age and serum galectin-3 (r=0.257; P<0.001), but not with sex (16). Interestingly, heart failure outpatients with galectin-3 values over the median serum concentration were also found to have lower hemoglobin values than those displaying galectin-3 values below this threshold (i.e., 82 vs. 89 g/L; P=0.004) (18), a finding that could not be confirmed in our healthy population. The data on the association between galectin-3 and BMI are also controversial. Unlike the study of Krintus et al., in which galectin-3 and BMI were found to be significantly associated in multivariate analysis (16), we observed a borderline significant association in univariate analysis (P=0.047) (Table 2), which however disappeared in multivariate analysis (P=0.305). All these differences with previous studies may be attributable to the different population studied (healthy subjects versus heart failure patients) or the different age of the subjects.
Conclusions
The results of our study suggest that the serum concentration of galectin-3 in middle-aged healthy individuals may be influenced only by renal function, but not by age, sex, BMI, liver function and hemoglobin concentration. Nevertheless, since all the serum galectin-3 values in our population were comprised within the currently accepted URL, the use of different cut-offs stratified according to the renal function seems unnecessary.
Acknowledgements
F Sanchis-Gomar is supported by a post-doctoral contract (APOSTD/2016/140) granted by the Regional Ministry of Education, Research, Culture and Sport (Valencia).
Footnote
Conflicts of Interest: The authors have no conflicts of interest to declare.
Ethical Statement: The evaluation was performed in agreement with the Declaration of Helsinki, under the terms of relevant local legislation, and was approved by the local Ethical Committee (University Hospital of Verona, Verona, Italy—SOPAV2, protocol number 35747; 25 July 2016). All subjects provided a written consent for being enrolled in this study.
References
- Benjamin EJ, Blaha MJ, Chiuve SE, et al. Heart Disease and Stroke Statistics-2017 Update: A Report From the American Heart Association. Circulation 2017;135:e146-e603. [Crossref] [PubMed]
- Sanchis-Gomar F, Perez-Quilis C, Leischik R, et al. Epidemiology of coronary heart disease and acute coronary syndrome. Ann Transl Med 2016;4:256. [Crossref] [PubMed]
- Sleight P. New methods for risk stratification in patients after myocardial infarction autonomic control and substrate sensitivity. J Am Coll Cardiol 2007;50:2291-3. [Crossref] [PubMed]
- Stillman AE, Oudkerk M, Bluemke D, et al. Assessment of acute myocardial infarction: current status and recommendations from the North American society for Cardiovascular Imaging and the European Society of Cardiac Radiology. Int J Cardiovasc Imaging 2011;27:7-24. [Crossref] [PubMed]
- Roffi M, Patrono C, Collet JP, et al. 2015 ESC Guidelines for the management of acute coronary syndromes in patients presenting without persistent ST-segment elevation: Task Force for the Management of Acute Coronary Syndromes in Patients Presenting without Persistent ST-Segment Elevation of the European Society of Cardiology (ESC). Eur Heart J 2016;37:267-315. [Crossref] [PubMed]
- Lippi G, Cervellin G. Risk assessment of post-infarction heart failure. Systematic review on the role of emerging biomarkers. Crit Rev Clin Lab Sci 2014;51:13-29. [Crossref] [PubMed]
- Salvagno GL, Pavan C. Prognostic biomarkers in acute coronary syndrome. Ann Transl Med 2016;4:258. [Crossref] [PubMed]
- Sanchis-Gomar F, López-Ramón M, Alis R, et al. No evidence of adverse cardiac remodeling in former elite endurance athletes. Int J Cardiol 2016;222:171-7. [Crossref] [PubMed]
- Sanchis-Gomar F, Bonaguri C, Aloe R, et al. Effects of acute exercise and xanthine oxidase inhibition on novel cardiovascular biomarkers. Transl Res 2013;162:102-9. [Crossref] [PubMed]
- Danese E, Lippi G, Montagnana M. Red blood cell distribution width and cardiovascular diseases. J Thorac Dis 2015;7:E402-11. [PubMed]
- Mueller T, Dieplinger B. Soluble ST2 and Galectin-3: What We Know and Don't Know Analytically. EJIFCC 2016;27:224-37. [PubMed]
- Lippi G, Cervellin G, Sanchis-Gomar F. Galectin-3 in atrial fibrillation: Simple bystander, player or both? Clin Biochem 2015;48:818-22. [Crossref] [PubMed]
- Lippi G, Mattiuzzi C. The biomarker paradigm: between diagnostic efficiency and clinical efficacy. Pol Arch Med Wewn 2015;125:282-8. [PubMed]
- Lippi G, Salvagno GL, Bonfanti L, et al. Relationship between serum galectin-3 values and demographical or biochemical variables in patients without acute coronary syndrome. Int J Cardiol 2014;171:270-1. [Crossref] [PubMed]
- Sanchis-Gomar F, Santos-Lozano A, Pareja-Galeano H, et al. Galectin-3, osteopontin and successful aging. Clin Chem Lab Med 2016;54:873-7. [Crossref] [PubMed]
- Krintus M, Kozinski M, Fabiszak T, et al. Establishing reference intervals for galectin-3 concentrations in serum requires careful consideration of its biological determinants. Clin Biochem 2017;50:599-604. [Crossref] [PubMed]
- Mueller T, Egger M, Leitner I, et al. Reference values of galectin-3 and cardiac troponins derived from a single cohort of healthy blood donors. Clin Chim Acta 2016;456:19-23. [Crossref] [PubMed]
- Stoltze Gaborit F, Bosselmann H, Kistorp C, et al. Galectin 3: association to neurohumoral activity, echocardiographic parameters and renal function in outpatients with heart failure. BMC Cardiovasc Disord 2016;16:117. [Crossref] [PubMed]
- Giannitsis E, Kurz K, Hallermayer K, et al. Analytical validation of a high-sensitivity cardiac troponin T assay. Clin Chem 2010;56:254-61. [Crossref] [PubMed]
- Gruson D, Mancini M, Ahn SA, et al. Galectin-3 testing: validity of a novel automated assay in heart failure patients with reduced ejection fraction. Clin Chim Acta 2014;429:189-93. [Crossref] [PubMed]
- Levey AS, Stevens LA, Schmid CH, et al. A new equation to estimate glomerular filtration rate Ann Intern Med 2009;150:604-12. [Crossref] [PubMed]
- D'Ascenzo F, Biondi-Zoccai G, Moretti C, et al. TIMI, GRACE and alternative risk scores in Acute Coronary Syndromes: a meta-analysis of 40 derivation studies on 216,552 patients and of 42 validation studies on 31,625 patients. Contemp Clin Trials 2012;33:507-14. [Crossref] [PubMed]
- Long B, Oliver J, Streitz M, et al. An end-user's guide to the HEART score and pathway. Am J Emerg Med 2017. [Epub ahead of print]. [Crossref] [PubMed]
- Turcato G, Serafini V, Dilda A, et al. Red blood cell distribution width at emergency department admission increases the accuracy of the HEART score for predicting death in patients with chest pain. Int J Cardiol 2016;222:999-1000. [Crossref] [PubMed]
- O'Donoghue ML, Morrow DA, Cannon CP, et al. Multimarker Risk Stratification in Patients With Acute Myocardial Infarction. J Am Heart Assoc 2016;5. [Crossref] [PubMed]
- Agnello L, Bivona G, Lo Sasso B, et al. Galectin-3 in acute coronary syndrome. Clin Biochem 2017. [Epub ahead of print]. [Crossref] [PubMed]
- French B, Wang L, Ky B, et al. Prognostic Value of Galectin-3 for Adverse Outcomes in Chronic Heart Failure. J Card Fail 2016;22:256-62. [Crossref] [PubMed]
Cite this article as: Danese E, Salvagno GL, Caruso B, Montagnana M, Sanchis-Gomar F, Lippi G. Physiologic determinants of serum galectin-3 in a general healthy population. Ann Res Hosp 2017;1:35.